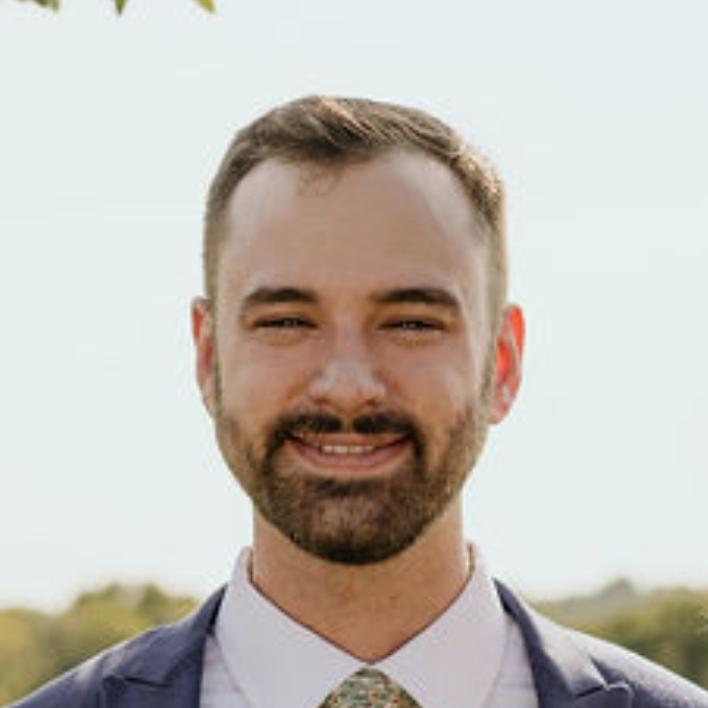
Hey I'm Matthew! I follow Jesus and work in aviation embedded systems software at Leidos. I previously worked as a Data Scientist in Baseball R&D @ Cleveland Guardians after finishing my Electrical Engineering PhD in 2023. From 2017-2022 I interned in spacecraft software development at NASA Johnson Space Center in Houston, TX.
I have two types of content here:
- Learning resources that tackle specific topics and are iteratively improved
- Blog posts that deal with specific example problems or tutorials
Posts
Is This A Bear?
How would you explain whether an image contains a bear? Maybe you’d point out the ears, the snout, or the color of the fur. But how would you come up with a number that quantitatively describes the “bearyness” of the image?
Autopicking Available SLURM Queues
I do a lot of my neural network training on a computing cluster at the University of Kentucky with GPUs. The cluster uses SLURM to submit jobs that run when enough resources (computer nodes) are available. This lets me run batches of experiments simultaneously and just watch the results from my desktop computer in the lab.
Launching Your Machine Learning Models with XGBoost(ers)
Recently, I was asked to pick and explain a predictive machine learning technique for a job application. One such technique is gradient boosted decision trees. I’ll introduce decision trees and then what “boosting” means and how “gradient” fits into this. I’ll use a baseball example of predicting a fielder’s putout rate given the direction, launch angle, and exit velocity of a batted ball and then return to it in more concise and less technical way at the end.
Yes, But What Exactly Is The Blockchain?
The blockchain is a list of transactions. However, this list is not stored in one common place but is copied across many computers, none of which are considered the “true” copy. Each computer keeps its own version of the list, so the list is distributed and no single person can assume control. The trick is keeping all the computers in sync.
Deep Landscaping
Deep learning can be split into three parts that must all work together to learn the desired task (like recognizing images, generating text, or detecting machine failures):
The Best Analysis of the Best First Guess in Wordle
There has been much ado across the Internet lately about the best first guess for Wordle. I can’t review all the proposed words, but a few include
roate
,arose
,soare
,later
, andadieu
.Let's Calculate a Lagrange Point For Fun
The James Webb Space Telescope needs to be consistently shielded from the light and heat of the Sun and that reflected by the Earth. Ultimately, we need two things:
Attention, But Explained Like You're A Normal Human
If you don’t have a deep learning background, everything about this will be confusing if it isn’t already.
Sorry.
Pro(ish) Tips On How To Code Machine Learning Models
With the amount of Python coding I do for machine learning, I have stumbled across some workflow tips that have greatly improved my standard of living. Check out these recommendations when developing your next breakthrough deep learning model.
You Should Use ISO 8601
Consider the following from popular web comic XKCD:
Making Sense of the Embedded Landscape
The world of embedded hardware and firmware is confusing.
Markov Chain Monte Carlo Sampling
One of my courses recently introduced Markov Chain Monte Carlo (MCMC) sampling, which has a lot of applications. I’d like to dive into those applications in a future post, but for now let’s take a quick look at Metropolis-Hastings MCMC.
subscribe via RSS